Election forecasting has evolved into a critical aspect of modern politics, shaping how the public, media, and political parties perceive electoral outcomes. In the United Kingdom, where elections can be fiercely contested, accurate predictions are more valuable than ever. With every general election, local election, or referendum, the ability to gauge likely outcomes through data-driven analysis becomes a vital part of understanding the political landscape. At the heart of this process is the science of election forecasting, which relies on a blend of polling data, statistical models, historical patterns, and sophisticated algorithms. This article explores how these various elements come together to create predictions that can often seem almost prophetic.
The Foundation: Polling Data
Polling data serves as the cornerstone of election forecasting. Public opinion polls, conducted by various organizations, offer a snapshot of how voters feel about political parties, leaders, and key issues at a given moment. These polls provide the raw data from which forecasts are derived. Pollsters typically collect data through various methods, including phone surveys, online questionnaires, and face-to-face interviews. Polls aim to capture a representative sample of the population, and when conducted rigorously, they can offer valuable insights into voter intentions.
However, polls are not perfect. They are susceptible to several sources of error, including sampling bias, response bias, and timing issues. For example, a poll taken months before an election may not account for late shifts in voter preferences. Pollsters must also ensure that their sample is representative of the electorate, which can be challenging given the diverse demographics and regional variations in the UK.
Despite these challenges, polling data remains an indispensable part of election forecasting. The key to turning poll results into accurate predictions lies in how forecasters analyze and adjust the raw data.
The Art of Adjusting Poll Data
To improve the accuracy of polls, election forecasters make several adjustments. One common adjustment is weighting, which ensures that the poll sample reflects the demographic makeup of the electorate. For example, if a poll includes too many respondents from a particular age group or socioeconomic background, the results may be skewed. By applying weights to the data, pollsters can correct for these imbalances and produce more reliable estimates.
Another important adjustment involves accounting for “shy voters”—those who may be reluctant to admit their true voting intentions. This phenomenon was particularly evident in the 2015 UK General Election, when polls underestimated the Conservative Party’s support. Forecasters must consider the possibility of social desirability bias, where respondents may give answers they believe are more socially acceptable rather than what they truly believe.
Additionally, election forecasters often aggregate data from multiple polls to reduce the margin of error. Known as “poll averaging,” this method combines results from different polling organizations to smooth out anomalies and provide a more consistent picture of voter preferences.
Building Predictive Models
Once polling data is adjusted and aggregated, election forecasters move on to building predictive models. These models are mathematical frameworks that use polling data, historical trends, and various other factors to estimate the probability of different election outcomes. A well-designed model not only predicts which party is likely to win but can also offer insights into vote shares, seat distributions, and the likelihood of coalition governments.
Predictive models are built using statistical techniques like regression analysis, which examines relationships between variables. In election forecasting, these variables may include factors such as economic conditions, incumbency, party leadership, and regional dynamics. For example, if a party is performing poorly in polls but has a history of strong support in certain constituencies, a good model will account for this and adjust its predictions accordingly.
Some models also incorporate “fundamentals”—underlying factors that can influence election outcomes regardless of polling data. These fundamentals might include the state of the economy, government approval ratings, and even the political context of the election. For instance, elections held during times of economic downturns often see the ruling party struggle, while periods of economic growth may favor incumbents.
Incorporating Historical Patterns
Historical data is another vital component of election forecasting. By analyzing past elections, forecasters can identify trends and patterns that may help predict future results. For example, certain constituencies in the UK are considered “bellwether” areas, meaning their voting patterns tend to reflect the national result. Analyzing how these constituencies have voted in previous elections can provide clues about the likely outcome in an upcoming election.
Forecasters also examine voter turnout, which can have a significant impact on election results. In the UK, turnout tends to vary by region, age group, and political engagement. For example, younger voters are historically less likely to turn out in large numbers, but when they do, they often favor more progressive parties. By factoring in expected turnout rates, forecasters can refine their predictions.
In addition, the UK’s unique electoral system—First Past the Post (FPTP)—requires forecasters to consider how votes will translate into seats. Under FPTP, small changes in vote share can lead to significant shifts in seat distributions, particularly in closely contested constituencies. A party that wins a large number of seats by narrow margins may appear to “overperform” relative to its vote share, while another party may win fewer seats despite a strong showing in the popular vote.
The Role of Advanced Algorithms
In recent years, advances in computing power and data science have transformed election forecasting. Sophisticated algorithms, powered by machine learning, allow forecasters to analyze vast amounts of data quickly and efficiently. These algorithms can identify complex patterns and correlations that would be difficult for humans to detect. For example, machine learning models can predict how changes in voter sentiment on specific issues—such as immigration or healthcare—might influence overall voting behavior.
One of the advantages of machine learning is its ability to “learn” from new data. As more polling data becomes available in the run-up to an election, these algorithms can update their predictions in real time, providing increasingly accurate forecasts as election day approaches.
Limitations and Uncertainty
Despite all these advancements, election forecasting is not an exact science. The inherent uncertainty in human behavior means that no model can predict election results with absolute certainty. Unexpected events—such as scandals, international crises, or economic shocks—can cause rapid shifts in voter preferences that are difficult to anticipate. Furthermore, voter turnout is notoriously difficult to predict, and small errors in turnout estimates can lead to significant inaccuracies in forecasts.
That said, the goal of election forecasting is not to provide perfect predictions but rather to offer informed estimates based on the best available data. By understanding the science behind election forecasting, we can better appreciate the complexities and nuances involved in predicting electoral outcomes.
Conclusion
The science of election forecasting has come a long way, blending polling data, statistical models, historical analysis, and advanced algorithms to offer increasingly accurate predictions. While there will always be an element of uncertainty, modern election forecasting provides valuable insights into the dynamics of UK elections. As data science and technology continue to evolve, the accuracy and reliability of these predictions are only set to improve, making election forecasts an essential tool for political analysts, campaigners, and voters alike.
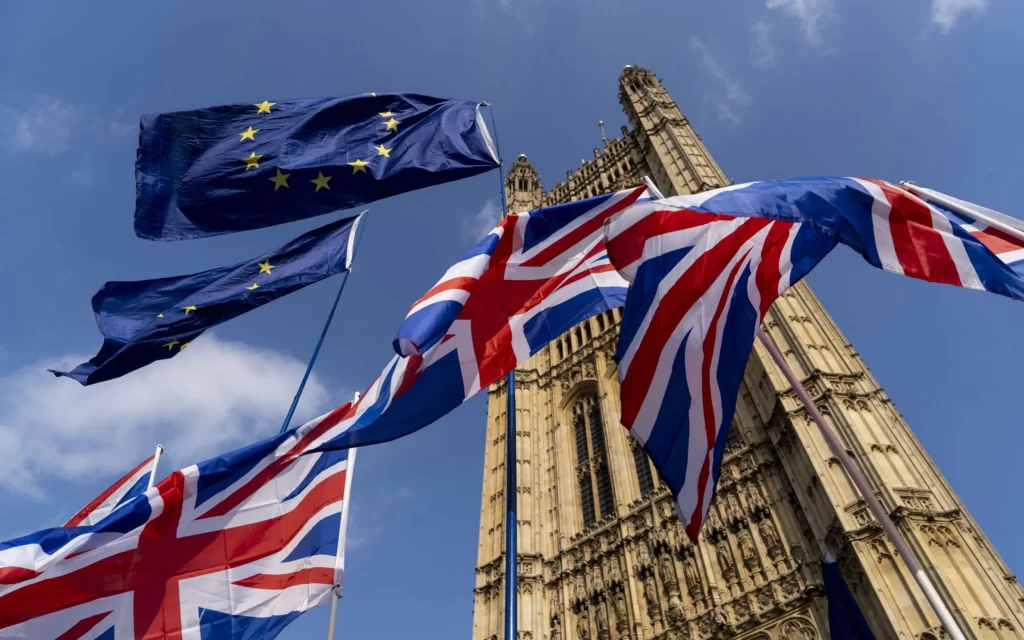